.jpg?w=1200)
Following a successful webinar conducted by Senior Software Architect Dr. Rasika Withanawasam that set in motion the LSEG Tech Talks series, organised by the London Stock Exchange Group (LSEG), Thayaparan Sripavan and Janaka Perera will be taking lead on the next webinar, focusing on ‘De-Risking the Risk with Machine Learning (ML) and Graphic Processing Units (GPUs)’ on August 20th.
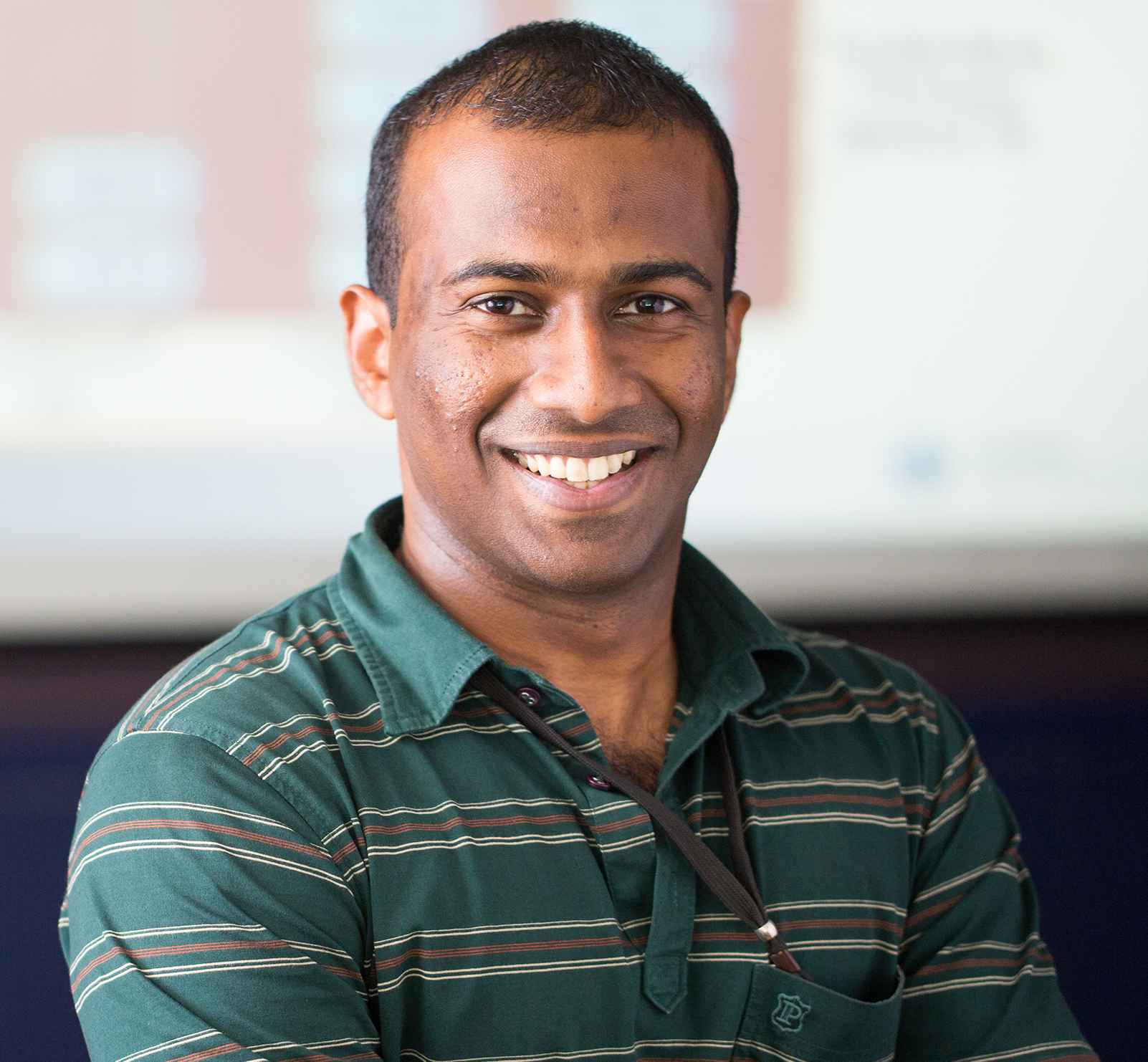
The webinar will be the second in the series of seven conducted by professionals and experts in the field of finance and technology, sharing their knowledge and expertise to educate the audiences on the themes impacting the global economy.
The Head of Hardware Accelerated Systems at LSEG, Thayaparan Sripavan joined the Group in 2005. As embedded systems had been a personal area of interest, it planted the seed to his experimenting with Field Programmable Gate Arrays (FPGAs) for the acceleration of financial systems a decade ago. Now with the full support of LSEG Technology, Thayaparan leads the efforts of designing and building hardware-accelerated hybrid solutions for the suite of capital market products offered by LSEG’s technology arm. They are now poised well for exploiting heterogeneous computing as it gets more standardised and accessible. He obtained his B.Sc. in Electronics and Telecommunication Engineering from the University of Moratuwa, and also holds an MBA from the Postgraduate Institute of Management, University of Sri Jayewardenepura.
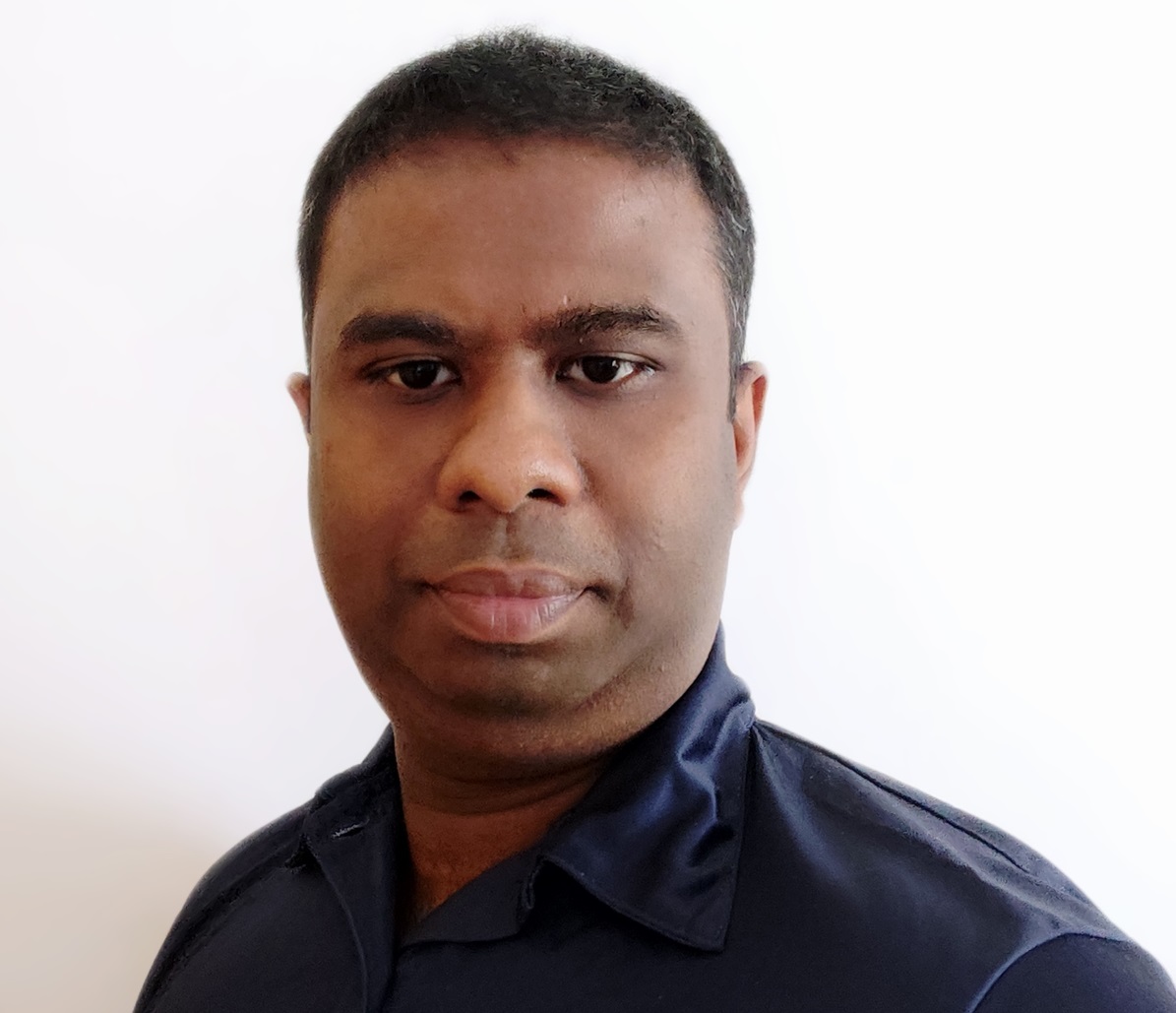
Janaka Perera is an Associate Architect in designing heterogeneous computing platforms for financial systems. Having been fascinated by the underlying hardware of computer systems, the way they work and digital electronics at a young age, Janaka mostly worked on software engineering before joining LSEG in 2017. Thereafter he began his career journey in heterogeneous computing, which allowed him to go into the depths of microarchitectures, which is a passion of his. He is responsible for exploring and architecting high-performance computing solutions in the fintech domain, making use of emerging technologies. His area of specialisation is data-parallel computing using General Purpose Graphics Processing Units.
Thayaparan and Janaka spoke with Roar, enlightening us on the topic of ML and GPUs along with financial risk management.
The topic the two of you will be covering will be ‘De-Risking the Risk with ML and GPUs’. Could you break it down for us?
We will be covering this topic from our domain perspective of improving financial risk management in capital markets, with some commonalities that are applicable to other sectors and problems as well.
Challenging the status quo requires a shift in the methodology (effectiveness) and the technology (efficiency).
We will delve into these twin aspects by discussing how ML (as a methodology) and General Purpose Graphics Processing Units (as a technology) address such a shift in financial risk management. We hope to highlight the importance, benefits, and challenges of being domain-specific in the application of ML using GPUs.
Can GPUs dramatically speed up computational processes when it comes to ML?
GPUs have come a long way since their inception, as they no longer just render graphics for general computing purposes; they can also support certain mathematical calculations and logic computation similar to a CPU.
With the use of GPUs, it is possible to accelerate the development of Artificial Intelligence systems because unlike a CPU, which has a limited number of core processors, a GPU has a massive number of processing cores, optimised for these kinds of applications. When we talk about Artificial Intelligence systems, there is no silver bullet to solve all the problems we may have. Therefore, when we craft models, they differ in nature based on the specific problems of interest, varying from how they work, the input and output. Such an approach is a journey that involves multiple iterations. GPUs help accelerate this phase significantly.
At LSEG, we mostly use NVIDIA Tesla range GPUs for ML and high-performance-computing-related problems. We also use GTX range GPUs outside work.
How can creating hardware-accelerated hybrid solutions accelerate performance for different algorithms, especially those used for ML?
General purpose computing is facing challenges as Moore’s law keeps pushing denser integrated circuits that need to be thermally efficient to be able to simultaneously use all of the available silicon estate. As a result, domain-specific hardware architectures have emerged, with Field Programmable Gate Arrays (FPGAs) entering the data centre, GPUs re-purposed for data-parallel computations, and Tensor Processing Units (TPUs) specifically for ML.
This trend can be expected to expand further, shifting away from ‘general purpose computing’ to what is referred to as ‘heterogeneous computing’, where dissimilar hardware architectures are used to efficiently solve computing problems specific to a domain. ML is a big early beneficiary of heterogeneous computing.
Do the benefits of incorporating ML and GPU into the financial sector outweigh the risks that have been addressed? To what extent do they mitigate the risks?
ML and GPUs bring certain possibilities that were prohibitive in the legacy world, a reality. We understand that existing systems have limitations. The existing approaches have certain limitations in how effective and efficient they can be in predicting risks of complex systems, quantifying the risk of complex systems, let alone mitigating it. There is essentially a gap between what exists today to where we would ideally want to be.
To bridge this gap, over time, technology has been evolving and businesses have been able to find smarter approaches. Today, it is addressed via human involvement, which brings gut-feel and different experiential perspectives into solving the issue.
Attempting to substitute or augment human intelligence with AI, is then a natural progression.
In risk management, the hardest to predict are the least recurrent, yet highly impactful adverse events. GPU-accelerated ML, as we see, could serve as an approach that would initially augment existing legacy approaches to bridge this gap.
How does Sri Lanka compare with the South Asian region in regard to bridging the gap between AI and human interaction? What are your personal solutions to bridging the aforementioned gap?
The easiest way to approach this is to begin using Artificial Intelligence to help humans make complex decisions effectively. This requires using AI in the right context. It is our view that a pre-packaged AI solution need not necessarily be the best for everyone. To realise this, Sri Lanka has a journey to go.
Which specific industries will benefit the most from ML and GPU?
AI can be beneficial for any industry where human experience together with knowledge plays the main role in the success of the industry. For high volumes of data where the algorithms allow parallel computing, GPUs not only save overall costs but allow faster computation or training time. At this point in time, there wouldn’t be any area of specialisation where one could conclude by saying that AI would not have any contributions in the future.
Interested in more of what Thayaparan and Janaka have to say on ML and GPUs? Sign up and participate in their webinar by simply clicking this link: https://lseg.zoom.us/webinar/register/WN_jGkDsRyJQ8WpijDX9gLYjA